Forced biased labeling in crowdsourced data, testing self-driving cars on past crashes, and more!
Last Week in AI #108
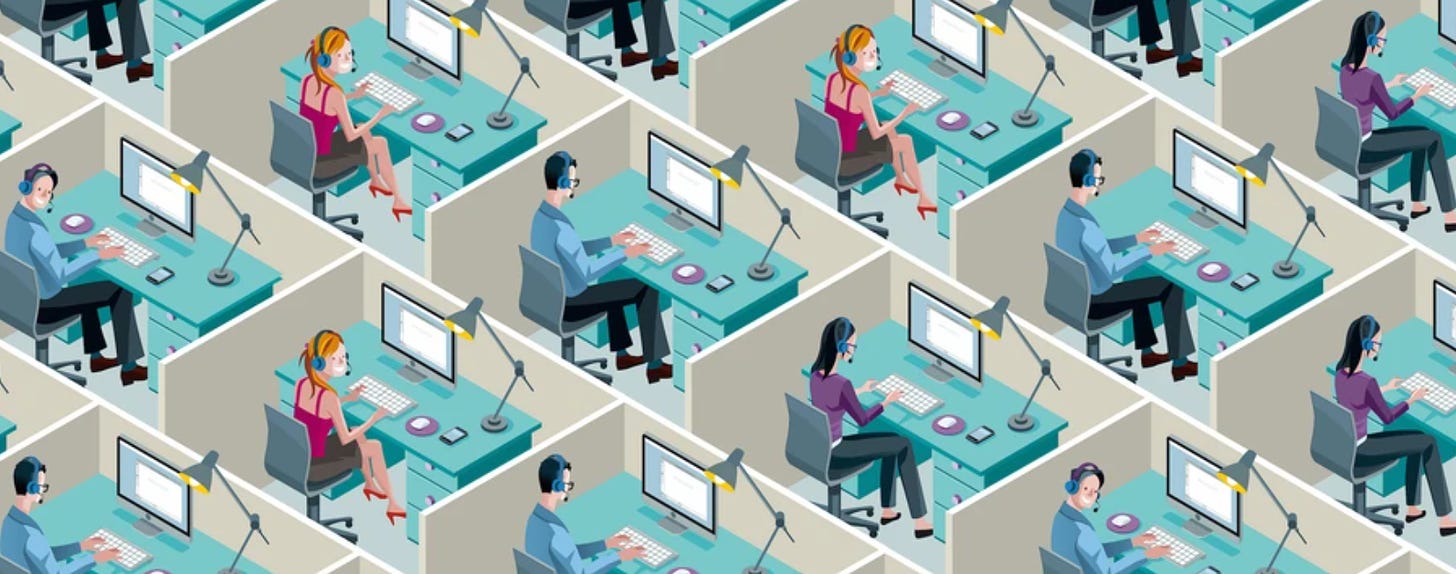
Mini Briefs
Underpaid Workers Are Being Forced to Train Biased AI on Mechanical Turk
Modern AI systems often require large datasets labeled by humans, and companies looking to leverage AI use online platforms, like Amazon's Mechanical Turk, to distribute data lableing tasks to remote workers. Such tasks can include rank…
Keep reading with a 7-day free trial
Subscribe to Last Week in AI to keep reading this post and get 7 days of free access to the full post archives.