Skynet This Week #5: Company news, weapons and bias concerns, AI history, and more!
Skynet This Week #5
By Andrey Kurenkov and Henry Mei
Our latest bi-weekly quick takes on a bunch of the most important recent media stories about AI, right in your email:
AI Advances & Business
Measuring abstract reasoning in neural networks
DeepMind blog
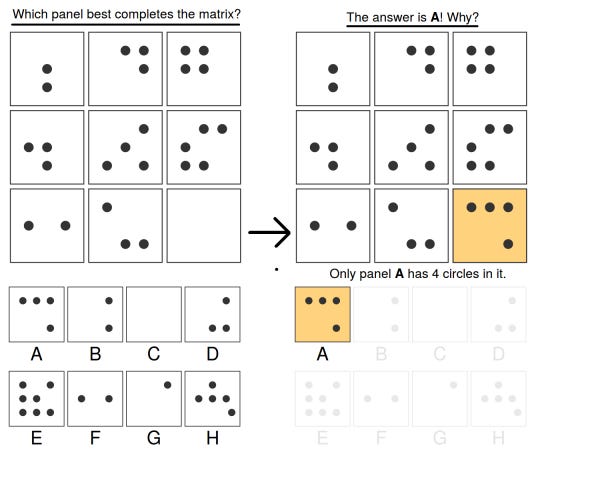
Credit: made with images from DeepMind's blog
A recent paper by DeepMind researchers suggested a new wa…
Keep reading with a 7-day free trial
Subscribe to Last Week in AI to keep reading this post and get 7 days of free access to the full post archives.