TL;DR: Recent advances have enabled AI models to transform text into other modalities. This article overviews what we’ve seen, where we are now, and what’s next.
Introduction
You’re reading text right now–it’s serving as a medium for me to communicate a sequence of thoughts to you. Ever since humanity became a band of degenerates that actually wrote things down instead of using their memories, we’ve been using sets of signs to transmit information. Under some definitions, you might call all of this “text.”
Today, and over the past centuries, we have encoded our knowledge of the world, our ideas, our fantasies, into writing. That is to say, much of human knowledge is now available in the form of text. We communicate in other ways too–with body language, images, sounds. But text is the most abundant medium we have of recorded communications, thoughts, and ideas because of the ease with which we can produce it.
When GPT-3 was fed the internet, it consumed our observations about the world around us, our vapid drama, our insane arguments with one another, and much more. It learned to predict next words in sequences of the tokenized chaos of human expression. In learning how we form sequences of words to communicate, a large language model learns to mimic (or “parrot”) how we joke, commiserate, command. GPT-3 kicked off something of a “revolution” by being extremely good at “text-to-text”: prompted with examples of a task (like finishing an analogy) or the beginning of a conversation, the generative model can (often) competently learn the task or continue the conversation.
There is almost a “universality” to the ways we use text, and we have only recently reached a point where AI systems can be put together in order to exploit how we use language to describe other modalities. The progress that enabled powerful text generation also enabled text-conditioned multimodal generation. “Text-to-text” became “text-to-X.”
In “text-to-text,” you could ask a model to riff on a description of a dog. In text-to-image, you could turn that description into its visual counterpart. Text-to-image models afforded a new ability not present in existing image generation systems. Existing models like GANs were trained to, given noise inputs (and class information for class-conditional image generation) generate realistic images. But these models did not offer the level of controllability that DALL-E 2, Imagen, and their ilk provide users: you could ask for a photo of a kangaroo with sunglasses, standing in front of a particular building, holding a sign bearing a particular phrase. Your wish was the algorithm’s command.
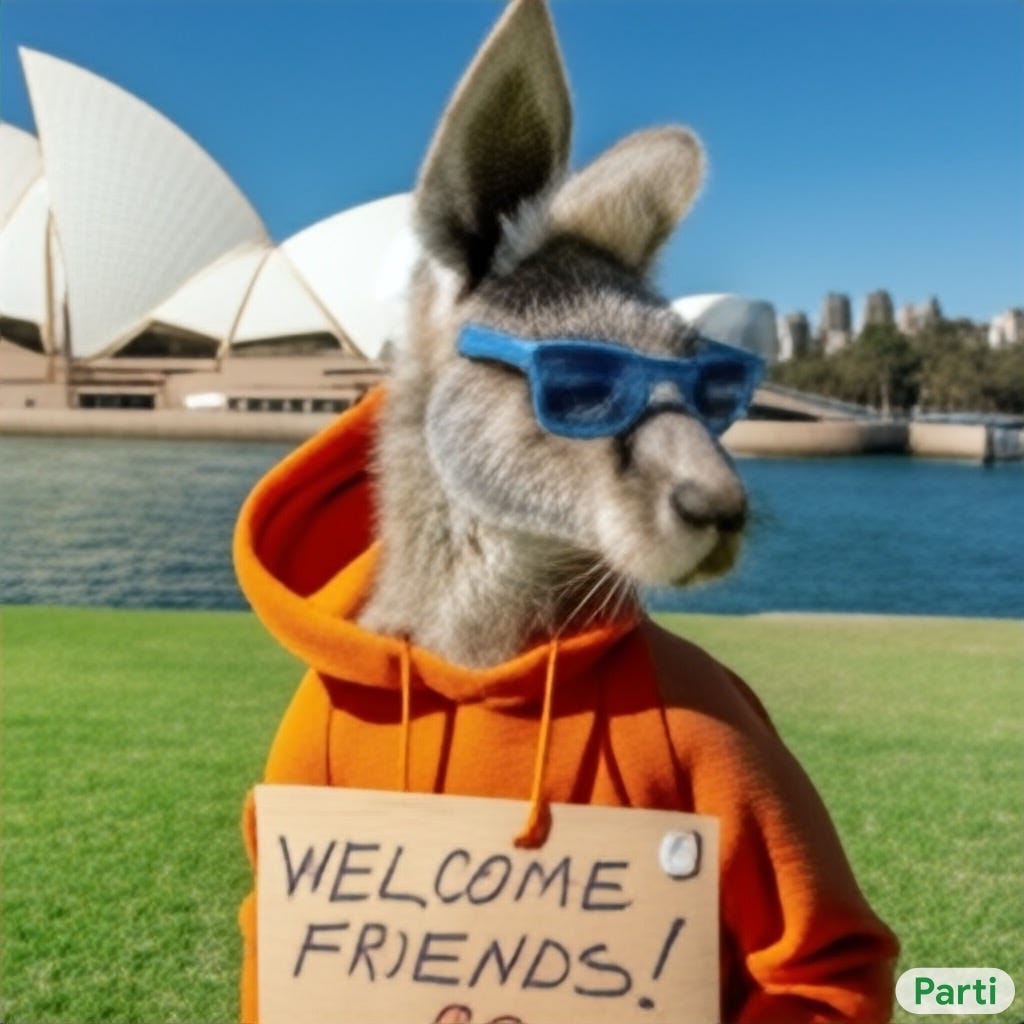
Keep reading with a 7-day free trial
Subscribe to Last Week in AI to keep reading this post and get 7 days of free access to the full post archives.